Harnessing AI/ML and Gen AI in Finance: Opportunities, Risks and Strategies
- Sameer Goyal
- Jan 16
- 4 min read
Updated: Jan 25
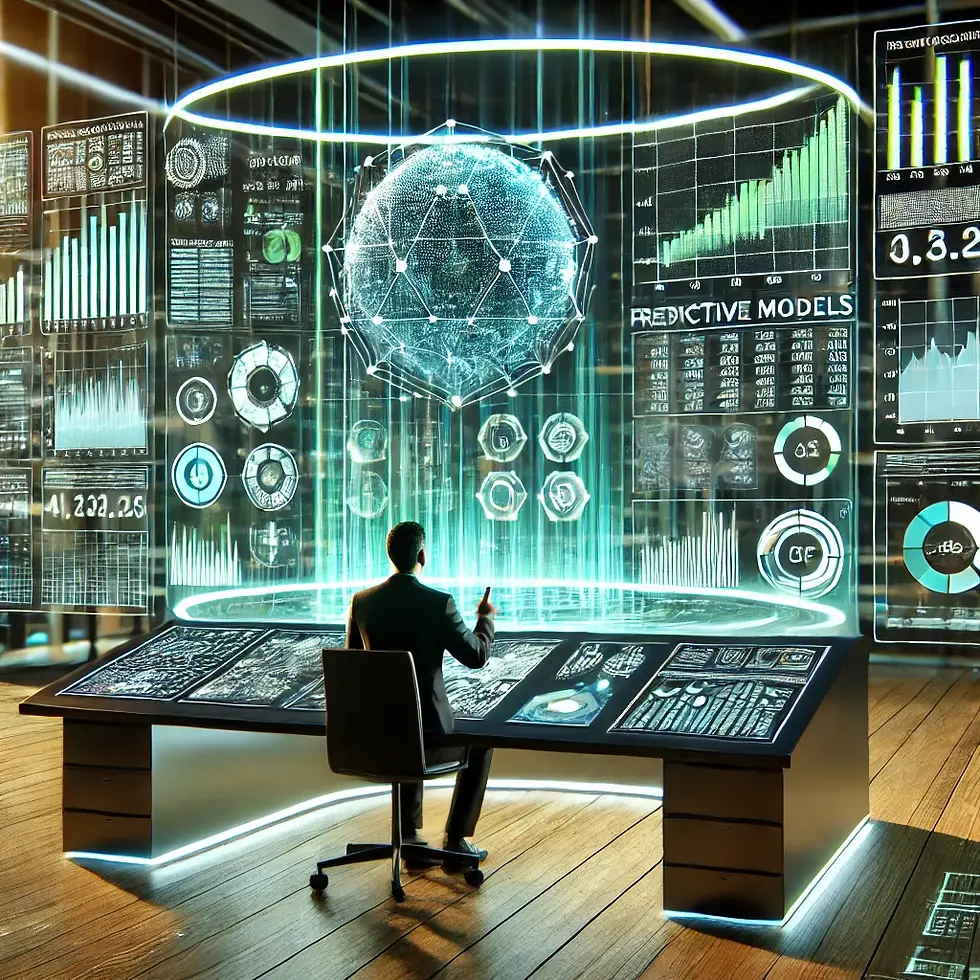
The financial services industry is undergoing a significant transformation, driven by advancements in Artificial Intelligence (AI), Machine Learning (ML) and Generative AI (GenAI). These technologies are not just buzzwords; they are reshaping how financial institutions operate, while offering new ways to innovate, improve efficiency and enhance customer experiences. However, with great power comes great responsibility. Let's explore how these technologies benefit the financial sector, analyse the associated risks and manage these risks while fostering innovation.
The benefits of AI/ML and GenAI
1. Improving customer experience
AI and ML are changing the way customers interact with financial services. AI-driven virtual assistants, such as Bank of America’s Erica or Capital One’s Eno – which are available 24/7 to help manage finances, track spending and provide personalised advice – not only make life easier for customers, but also help banks reduce costs.
GenAI takes personalisation a step further by offering tailored investment recommendations based on individual financial goals and risk tolerance, making financial advice more targeted and relevant.
2. Detecting frauds and managing risks
AI and ML are incredibly effective at spotting fraudulent activities by analysing vast amounts of transaction data in real time. These systems can detect unusual patterns and flag potential frauds much faster than traditional methods. For instance, JPMorgan Chase uses AI to monitor transactions and prevent frauds, saving millions.
GenAI can also help manage risk by simulating different financial scenarios and stress-testing models. This allows financial institutions to anticipate market disruptions and devise proactive strategies.
3. Boosting operational efficiency
AI and ML can automate repetitive tasks, freeing up human resources for more strategic activities. This includes automating processes such as loan approvals, customer onboarding and compliance checks. HSBC, for instance, leverages AI to streamline its compliance processes, significantly reducing time and costs.
GenAI can further enhance efficiency by automating the creation of financial reports and documents, ensuring accuracy and consistency while saving time.
4. Enhancing decision-making
AI and ML provide robust tools for data analysis and decision-making. By analysing historical data and market trends, AI offers insights that inform investment strategies and risk assessments. BlackRock’s Aladdin platform, for instance, uses AI to analyse market data and optimise investment portfolios.
GenAI can assist in decision-making by generating predictive models and scenarios, helping financial professionals make more informed choices, especially in areas such as asset management and financial planning.
The risks and challenges
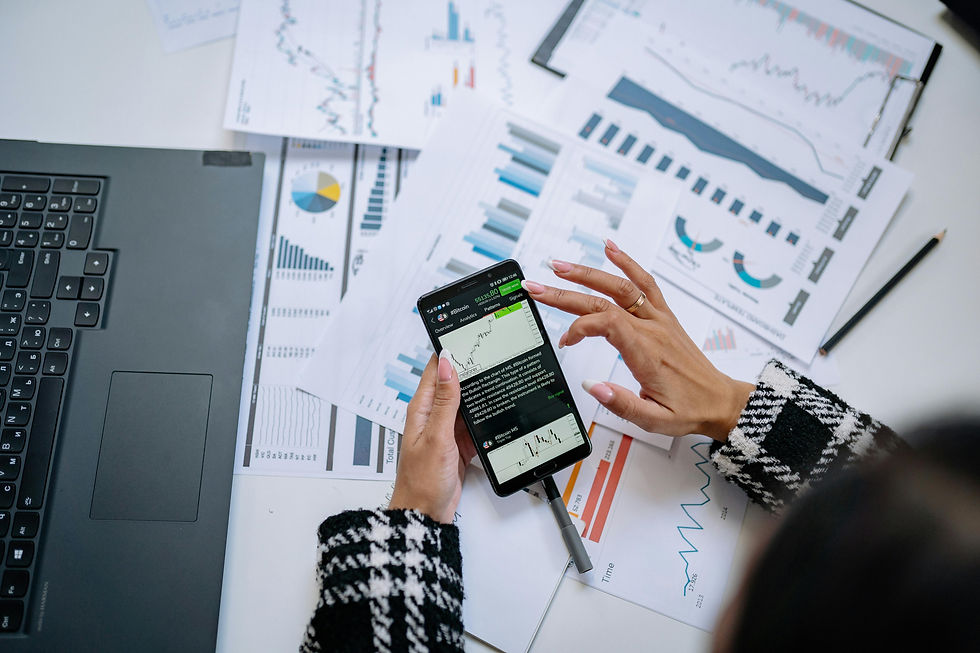
1. Data privacy and security
Handling large volumes of sensitive financial data with AI/ML and GenAI raises significant privacy and security concerns. Financial institutions must ensure AI systems comply with data protection regulations and implement robust cybersecurity measures to prevent data breaches.
2. Bias and fairness
AI algorithms can unintentionally perpetuate biases present in training data, leading to unfair outcomes. For example, biased credit scoring models can result in discriminatory lending practices. Regular auditing of AI systems is essential to identify and mitigate such biases.
3. Explainability and transparency
AI/ML models, especially deep learning algorithms, often operate as "black boxes," making it difficult to understand their decision-making processes. This lack of transparency can be problematic, particularly in regulatory environments where explainability is required. Financial institutions must invest in developing explainable AI models and ensure transparency of their decision-making processes.
4. Regulatory compliance
The rapid adoption of AI/ML and GenAI in financial services has outpaced regulatory frameworks, creating uncertainty and potential compliance risks. Financial institutions must stay updated with evolving regulations and work closely with regulators to ensure their AI systems meet compliance requirements.
Mitigation strategies
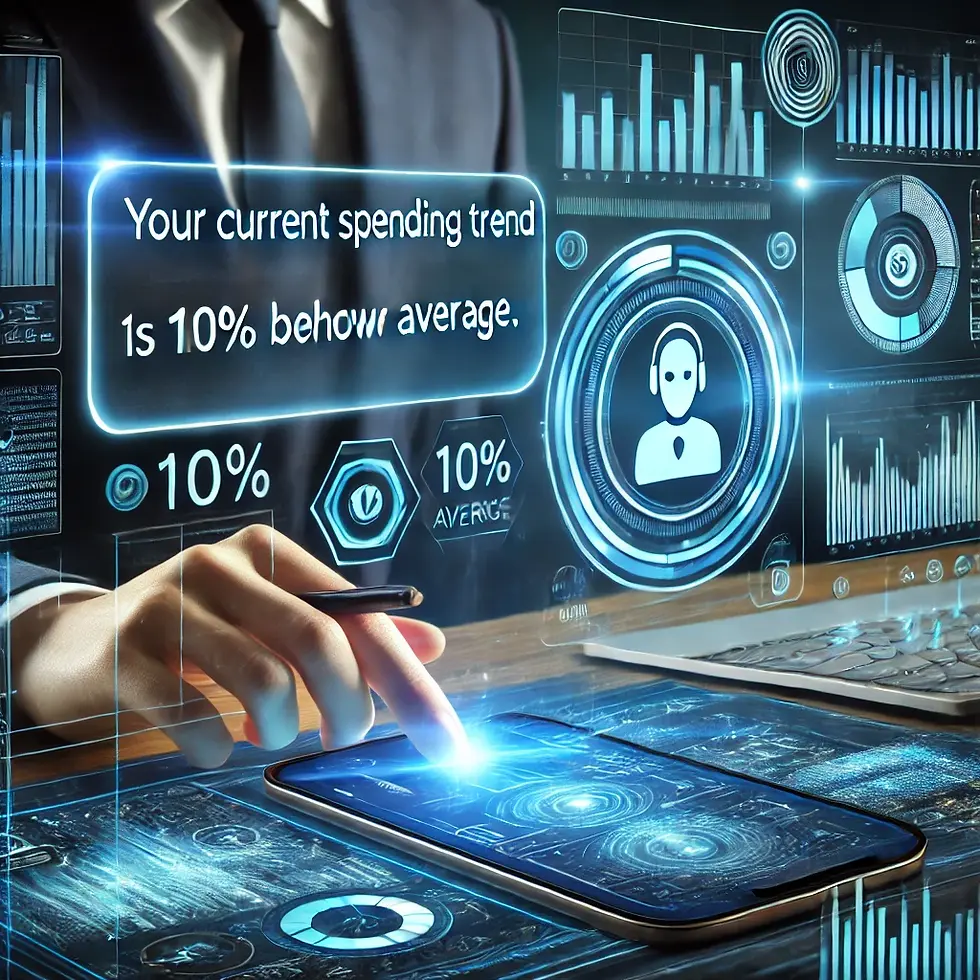
1. Strong data governance
Implementing robust data governance frameworks is essential to ensure data quality, privacy and security. Financial institutions should implement clear policies for data collection, storage and usage, alongside advanced encryption techniques to protect sensitive information.
2. Bias mitigation techniques
To address bias, financial institutions should use diverse and representative datasets for training AI models. Regular audits and fairness assessments can help identify and mitigate biases. Techniques such as adversarial debiasing and fairness constraints can further enhance model fairness.
3. Explainable AI
Investing in explainable AI technologies can help demystify complex AI models. Techniques such as Local Interpretable Model-agnostic Explanations (LIME) and SHapley Additive exPlanations (SHAP) can provide insights into how AI models make decisions, enhancing transparency and trust.
4. Collaboration with regulators
Financial institutions should proactively engage with regulators to shape the development of AI regulations. Collaborative efforts can help ensure alignment of regulatory frameworks with technological advancements and that compliance requirements are clear and achievable.
5. Continuous monitoring and auditing
Regular monitoring and auditing of AI systems is crucial to ensure their ongoing performance and compliance. Financial institutions should establish dedicated AI governance teams to oversee the deployment and operation of AI models, ensuring their compliance to ethical standards and regulatory requirements.
Encouraging innovation and transformation
Despite the risks, the potential benefits of AI/ML and GenAI in financial services are immense. By adopting a balanced approach that emphasises both innovation and risk management, financial institutions can harness the power of these technologies to drive transformation and achieve sustainable growth.
To foster innovation, financial institutions should invest in research and development, collaborate with technology partners and participate in industry consortia. Encouraging a culture of experimentation and learning can help institutions stay ahead of the curve and continuously improve their AI capabilities.
In conclusion, AI/ML and GenAI offer transformative opportunities for the financial services industry, from enhancing customer experiences to improving risk management and operational efficiency. However, these technologies also pose significant risks that must be carefully managed. By implementing robust data governance, bias mitigation techniques, explainable AI and proactive regulatory collaboration, financial institutions can navigate the challenges and unlock the full potential of AI/ML and GenAI, driving innovation and transformation in the industry.
(The author is Head of Engineering- BEAT, Acuity Knowledge Partners. Views expressed are personal.)
Comments