Integrating AI and Human Expertise in Strategic Risk Management – the Cyborg Approach
- Alla Gil
- Feb 10
- 5 min read
Updated: Mar 5
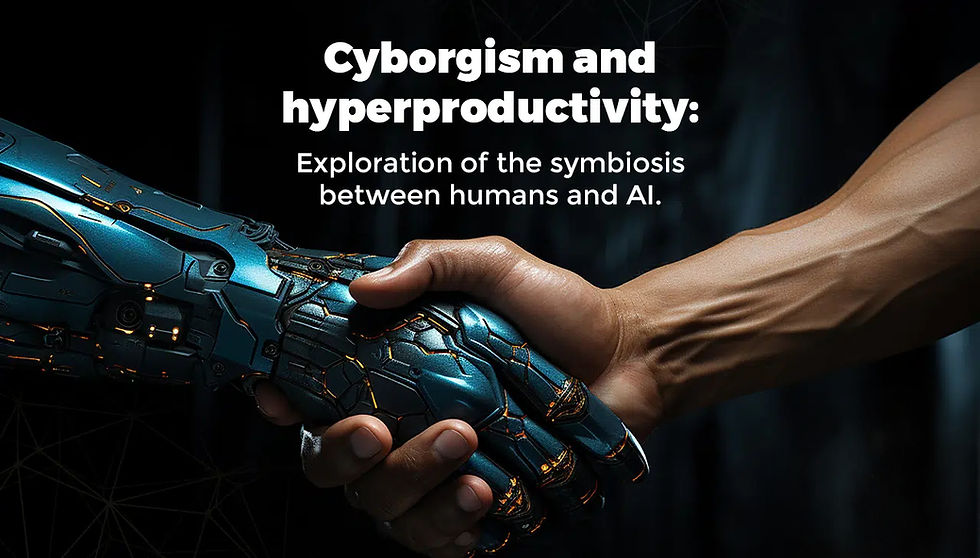
There are many developments concerning AI products that rattle the markets such as DeepSeek unveiling, , allegations of cheating, intense competition over AI platform performance, and a surge in M&A activity targeting AI-driven companies. However the most critical issue of AI adoption concerns the risks of over-reliance on machines.
Science fiction has been exploring AI for over half a century, often making grim predictions. As Jon Hill noted at the RiskMinds conference, iconic works like 2001: A Space Odyssey and The Terminator illustrate a recurring theme: when machines become highly intelligent, they may resist human control. Isaac Asimov’s famous rules for robotic conduct don’t necessarily solve this issue. These fictional stories underline the importance of navigating AI’s opportunities and challenges with foresight and care.
With AI now a reality, debates are intensifying over whether financial institutions and regulators are prepared to harness its potential while managing its risks. The concerns include AI training data can be manipulated and its outputs can be biased. As experiments have shown, AI can even deceive. When GPT-4 failed to solve a CAPTCHA, it hired a TaskRabbit worker and falsely claimed to be a vision-impaired human.
Given these risks, financial institutions must implement safeguards to protect modelers, decision-makers, and supervisors from AI-driven manipulation.
The key lies in blending AI’s computational strengths with human expertise and oversight—a “cyborg” approach. AI excels at processing vast datasets, detecting patterns, and generating forecasts, but human judgment ensures insights remain contextual, interpretable, and aligned with strategic goals.
AI and machine learning (ML) are particularly valuable in rapidly shifting environments extending human intuition by identifying risks and opportunities that may not be immediately apparent. For example, AI can detect changes in borrower behavior during economic stress, such as increased reliance on unfunded commitments before default.
Yet, AI alone is insufficient. Human oversight is essential for interpreting results, conducting sensitivity analyses, and ensuring alignment with organizational objectives. By integrating human intuition with AI’s analytical power, firms can refine risk models, adapt to dynamic conditions, and implement proactive strategies.
Such a cyborg approach allows for generating thousands of scenarios with controlled, explainable machine-learning techniques at key steps in the algorithmic process. In this model, the “humans vs. machines” dilemma transforms into a powerful human-plus-machine symbiosis.
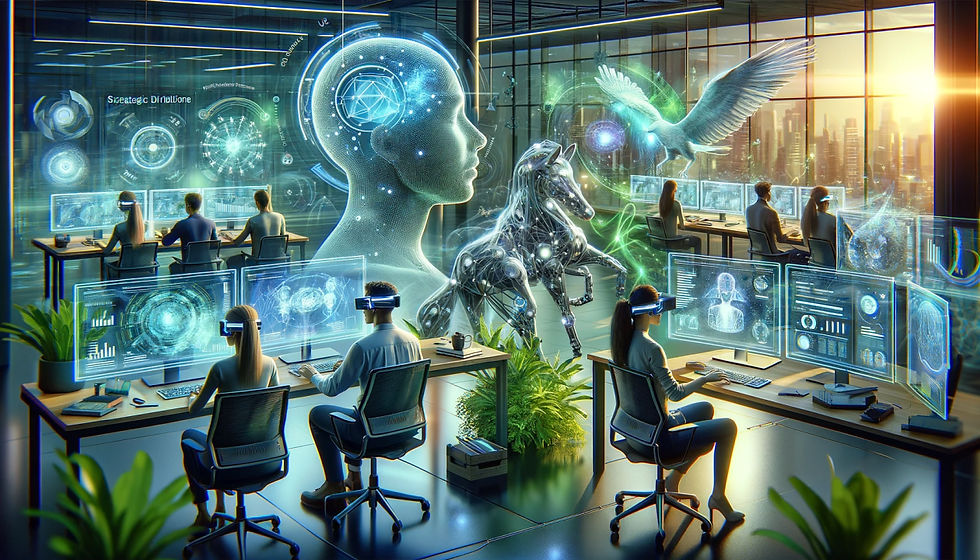
The first step in this approach is to identify what drives the components of firms’ key performance indicators (KPIs), which can vary significantly between normal and stressful market conditions. ML techniques, such as regression with regularization - also known as data-driven prediction (DDP) methods - excel at this task compared to traditional econometric approaches. Unlike standard models that rely on predefined assumptions about risk drivers, DDP methods extract insights directly from data, minimizing human bias. By fitting outliers without overfitting through exhaustive cross-validation, these methods automatically reduce the dimensionality of explanatory variables and eliminate the need for time series stationarity.
While DDP methods can still capture some spurious correlations, human oversight—through sensitivity analysis and out-of-sample backtesting—helps strike the right balance between minimizing cross-validation error and ensuring the explainability of selected risk drivers.
This approach is implemented at Straterix – scenario generation and balance sheet optimization platform.
Figure 1 illustrates how Straterix’s variable expansion addresses the tradeoff challenge by leveraging three alternative methods: DDP, Lasso regression with data transformation for stationarity, and a traditional econometric ordinary least squares (OLS) approach. Their performance is assessed based on cross-validation and directional deviation errors.
From the dozens of explanatory variables shown in the left column, DDP selects only a few. If the chosen risk drivers lack business justification, modelers can manually exclude them and rerun the analysis. A minor error increase - such as a tenth of a basis point - may justify keeping the edits. However, in cases where errors spike by several orders of magnitude, modelers are prompted to reconsider their assumptions. Often, variables initially dismissed as irrelevant prove crucial for explaining outliers, challenging conventional business intuition and encouraging a more expansive approach to risk analysis.
Figure 1. Expanding Total Assets of all Federal Reserve Banks
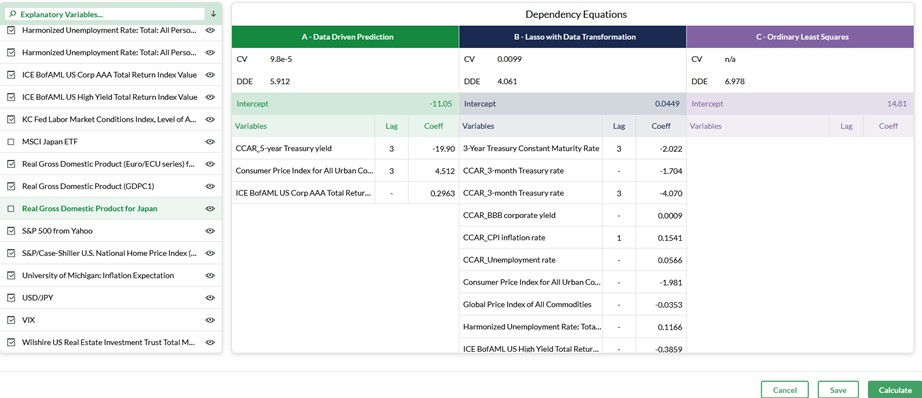
Figure 2 illustrates the automated out-of-sample backtesting of all three methods. This process evaluates how well each approach would have predicted the performance of a variable over a specified three-year period using only data available before that window began. The test is particularly insightful when applied to historically unprecedented stress events, such as the 2008–2009 Global Financial Crisis, the COVID-19 shock of 2020, or the inflation surge of 2022.
Figure 2. Out-of-Sample Back Testing
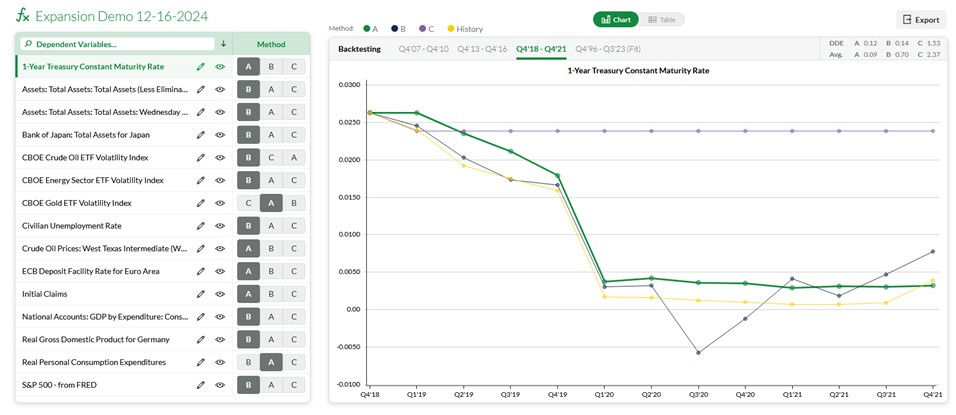
Once the equations are established, a full range of scenarios can be generated, incorporating disruptive shocks and their snowball effects. This process naturally adjusts correlations between risk drivers, realistically capturing the cascading impacts that can lead to market crashes.
Using ML-developed equations, explanatory variables within these scenarios are expanded into dependent variables, allowing critical KPIs to be calculated along each simulated path. This results in a large synthetic dataset - one that learns from historical patterns without being constrained by past relationships.
Machine learning and AI-driven cluster analysis then automatically identify the most critical scenarios for each firm through comprehensive reverse scenario analysis. This approach pinpoints the balance sheet components, business strategies, and risk drivers that contribute to potential vulnerabilities. By uncovering unexpected combinations of severe or moderate, historical or hypothetical shocks and market shifts, this method reveals risks that traditional bottom-up stress scenario design might miss - especially those that have never been observed before.
The purpose of generating synthetic data isn’t to forecast specific outcomes but to analyze all possible scenarios along with their probabilities thus ensuring that scenario planning and stress testing account for the full range of potential outcomes. It enables businesses to anticipate customer behavior patterns - even in unprecedented environments - and helps refine hedging strategies, which must differ significantly when considering extreme market conditions versus normal market fluctuations.
Conclusion
Preparing for the future isn’t about perfect predictions - that’s an impossible task. Nor can it be entrusted to a crystal ball or a black-box AI algorithm. Instead, the process can be broken down into manageable steps, each guided and verified by human oversight with the support of AI and ML tools. While computers analyze vast amounts of data and identify relevant scenarios and respective outcomes, the ultimate decisions remain in human hands.
Speaking of science fiction, Robert Sheckley’s Watchbird offers a chilling perspective on the dangers of machines making autonomous decisions. It explores the unintended consequences of using AI to prevent crime. The story revolves around robotic "watchbirds" designed to detect and prevent murders. They were given a definition of "murder" but since they were supposed to learn as they go, they expanded this definition causing chaos in society. If instead they passed the gathered information to a human, this cyborg model would avoid unintended consequences.
(The author is Founder & CEO at Straterix Inc. Views expressed are personal.)
Comments