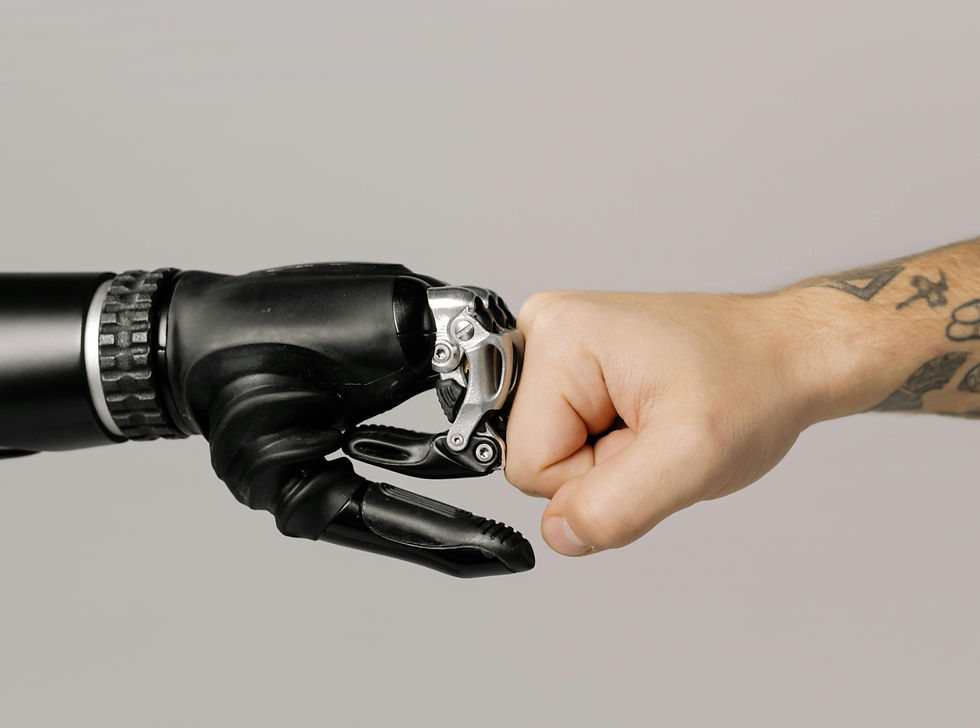
The Monetary Authority of Singapore (MAS) has just released a comprehensive Information Paper on Artificial Intelligence Model Risk Management (AI MRM). With financial institutions increasingly integrating AI into their operations—including the rapidly evolving generative AI technologies—this guidance arrives at a critical juncture. MAS’s key message is clear: while AI offers immense opportunities to drive innovation, improve efficiency, and enhance customer experiences, it also brings significant risks that require deliberate management.
In this article I try to offer an overview of MAS’s key recommendations, shedding light on what banks should prioritize—particularly in governance, model inventories, validation, and adversarial testing. Additionally, we compare MAS’s approach with that of the UK’s Prudential Regulation Authority (PRA) and reflect on MAS’s role as both a regulatory leader and an enabler of innovation.
A Structured Framework for AI Model Risk Management
MAS emphasizes that AI risk management must be deeply embedded within an institution’s existing governance, compliance, and operational risk frameworks. Its Information Paper outlines four key pillars:
Governance and Oversight: Establish clear accountability, update policies, and invest in cross-functional forums to manage AI-specific risks.
Robust AI Inventories: Maintain centralized records of AI models, documenting their lifecycle, purpose, and interdependencies to ensure transparency and oversight.
Validation and Ongoing Monitoring: Expand validation teams and implement regular testing protocols to keep models aligned with their intended goals.
Rigorous Development and Deployment Standards: Enforce high standards for data quality, explainability, fairness, and reproducibility to build trust in AI systems.
Governance and Oversight: Strengthening Accountability
AI risk management cannot be treated as an afterthought. MAS advises financial institutions to integrate AI considerations into their existing governance structures. This involves establishing cross-functional forums that bring together experts in compliance, risk, technology, and business operations.
Updating policies to reflect AI-specific concerns—such as fairness, accountability, and ethics—is also essential. Training programs tailored to equip staff with AI expertise can further enhance an organization’s ability to manage emerging risks effectively.
Building a Comprehensive Model Inventory: The Cornerstone of AI Governance
A standout recommendation from MAS is the emphasis on maintaining a centralized AI model inventory. This inventory should provide a clear picture of every AI model in use, documenting its purpose, data inputs, interdependencies, and performance across its lifecycle.
Such an inventory does more than ensure regulatory compliance. It serves as a proactive risk management tool, enabling institutions to:
Track model drift or unauthorized usage,
Understand dependencies between models, and
Identify risks before they escalate.
In our view, investing in modern inventory systems with automated tracking capabilities will be crucial for banks looking to meet MAS’s expectations.
Validation and Continuous Monitoring: Ensuring Stability
AI models, particularly those used in critical financial applications, require constant vigilance. MAS underscores the need for strong validation teams capable of probing models for weaknesses and ensuring they remain aligned with business objectives. Techniques like adversarial testing—where teams intentionally attempt to “break” a model—are vital to uncover hidden vulnerabilities.
For high-risk models, MAS recommends independent validation by teams separate from those involved in development. This is in any case in-line with best practice in the industry, starting from the days of the US SR 11-7 guidelines that came out in 2011. Continuous monitoring, supported by predefined metrics and thresholds, is essential to address risks like performance drift and unintended biases
Development and Deployment: Raising the Bar
MAS’s paper highlights the importance of stringent standards in AI development. From ensuring datasets are unbiased and well-documented to implementing explainability tools, financial institutions must focus on transparency and accountability at every step. Fairness checks for high-impact use cases further ensure that AI models do not inadvertently reinforce systemic inequalities.
By embedding these practices into development workflows, banks can bolster both the reliability and the credibility of their AI systems.
Generative AI: Balancing Opportunity with Caution
Generative AI holds immense potential, offering applications like natural language processing, code generation, and predictive analytics. However, MAS highlights significant challenges:
Unpredictable Outputs: Generative models can sometimes “hallucinate” results or amplify biases.
Testing Complexities: The lack of standard benchmarks complicates model evaluation.
Data Security Risks: Safeguards, such as private clouds and input-output filters, are essential to prevent sensitive data leakage.
MAS advises financial institutions to approach generative AI cautiously. Early-stage deployments should focus on non-critical applications, with robust monitoring and controls in place.
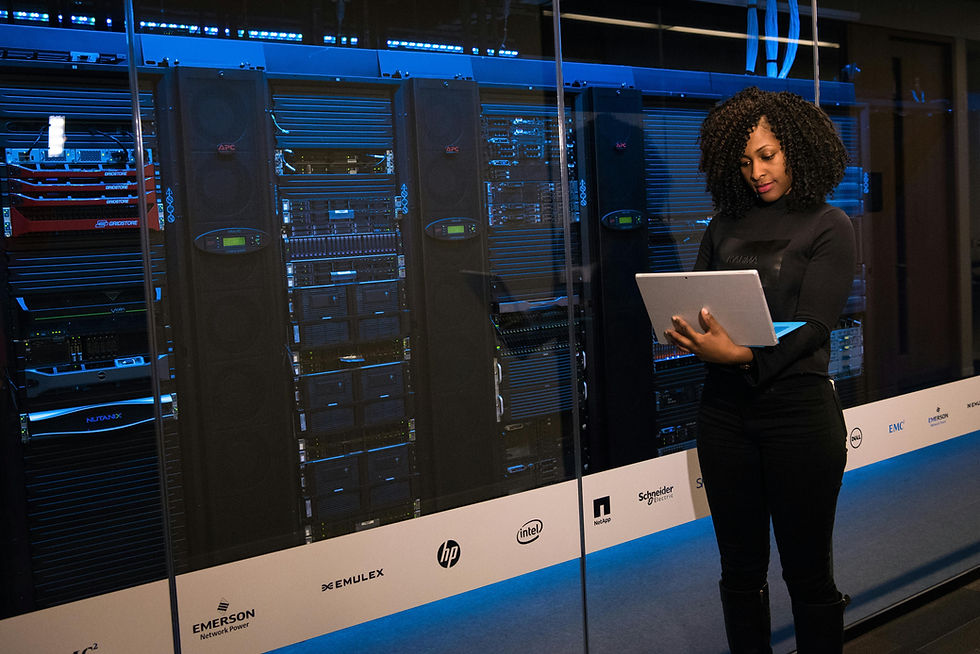
How does this report compare with AI model risk management guidance under UK PRA SS 1/23?
In 2023, the UK’s Prudential Regulation Authority (PRA) issued Supervisory Statement (SS) 1/23, addressing similar concerns about AI model risk management. While both MAS and PRA stress governance, validation, and monitoring, MAS’s Information Paper stands out for its operational focus. Recommendations like centralized model inventories and lifecycle tracking provide financial institutions with practical steps to navigate AI risks.
This granular approach could potentially set the standard for future regulatory guidance that may come from other regulators subsequently.
MAS: Balancing Innovation with Prudence
Beyond its role as a regulator, MAS has been also seen as a champion of financial innovation. Its initiatives—such as regulatory sandboxes and sustainability-focused policies— were examples of fostering a forward-looking financial ecosystem. The Information Paper on AI MRM reflects this balance, addressing risks without stifling innovation. By focusing on AI early, in our opinion MAS is looking to ensure that financial institutions operating in Singapore remain geared up for the rapid advances in global financial technology.
What Banks Should Do Now
MAS’s guidance is not just about compliance—in our view it’s a call to action for banks to future-proof their AI practices. Key priorities include:
Building a Centralized Model Inventory: This investment will enhance visibility, oversight, and risk management.
Expanding and Upskilling Validation Teams: Expertise in AI-specific validation methods is critical to managing increasingly complex models.
Implementing Adversarial Testing and Monitoring: Proactive measures can identify weaknesses before they cause harm.
Embedding AI-Specific Standards Across the Organization: Fairness, explainability, and strong data management should be integral to every AI project.
Conclusion
MAS’s Information Paper on AI MRM offers a practical and timely framework for managing the risks associated with AI adoption in financial services. While the regulator deserves credit for its proactive and detailed approach, the paper’s greatest strength lies in its actionable guidance. By following these recommendations, financial institutions can position themselves not only as compliant but as leaders in responsible AI innovation—harnessing its transformative potential with confidence and care.
(The author is Managing Director of InCred Insight. Views expressed are personal.)
Comentários