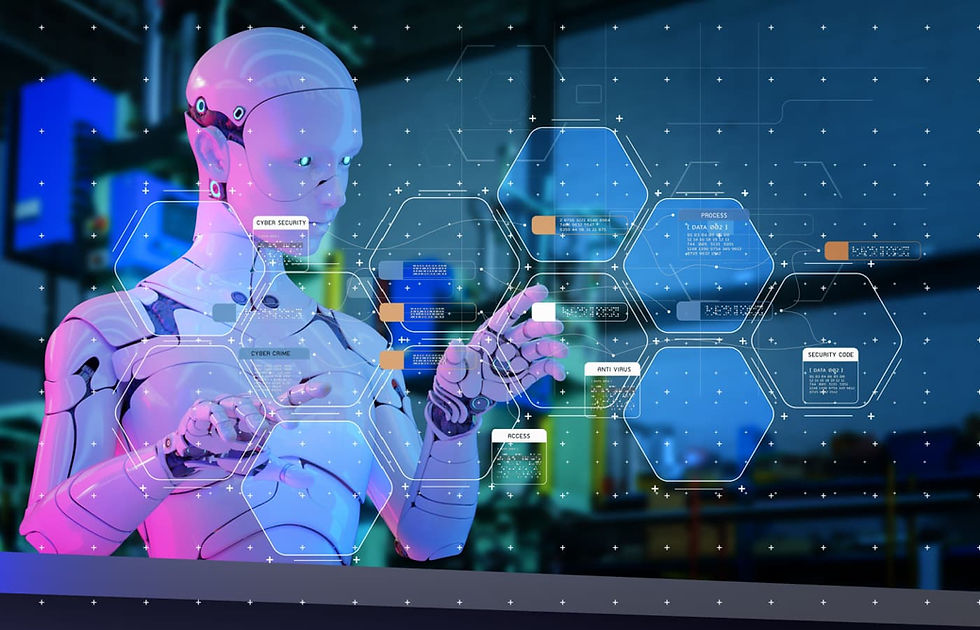
Financial crime detection has evolved dramatically over recent years, driven by advances in artificial intelligence (AI) and machine learning (ML). Banks and other financial institutions are now increasingly moving to cloud-native solutions to enhance their capabilities in combating financial crime. This shift is not only a response to the sophistication of modern threats but also reflects a broader industry trend towards scalability, adaptability, and real-time insights provided by AI and ML. The use of cloud-native AI solutions is seen as a transformative move, especially as established and emerging players are now offering specialized capabilities tailored to financial crime compliance.
The Financial Crime Landscape and Cloud-Native AI
Financial institutions are facing significant challenges due to the sheer volume of transactions and the complexity of criminal techniques. As traditional compliance measures often lack the flexibility to detect emerging threats effectively, institutions are now leveraging cloud-native machine learning models. These models bring real-time, scalable analytics that can quickly adapt to new typologies of financial crime, providing financial institutions with the agility needed to stay ahead of criminal activities.
Key cloud-native features provide institutions with automated and self-learning systems, which are integral to maintaining robust financial crime compliance programs. This real-time adaptability is particularly critical in today’s landscape, where transaction patterns and financial crime techniques evolve rapidly.
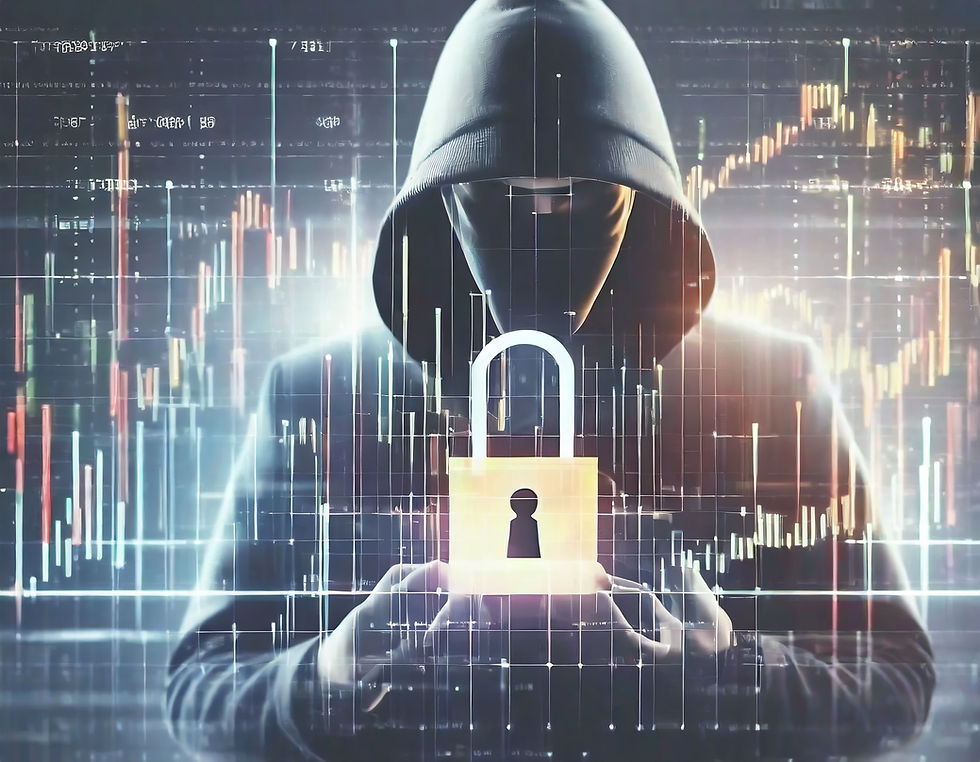
Technical Features of Cloud-Native Financial Crime Detection Solutions
A significant advantage of cloud-native solutions is their modular architecture, allowing for seamless scaling and integration. Modern financial crime platforms often utilize microservices, enabling specific components of the detection process (e.g., transaction monitoring, alert management) to be upgraded or replaced without disrupting the entire system. Many cloud-based platforms are also highly adaptable, providing integration capabilities with other AML and compliance systems.
Machine Learning Algorithms and Automated Pattern Detection:
Cloud-native platforms leverage advanced ML algorithms to process and analyze vast amounts of structured and unstructured data. Solutions deploy both supervised and unsupervised learning models to uncover hidden relationships and detect patterns indicative of financial crime.
Feature engineering has become increasingly sophisticated, encompassing variables like behavioral patterns, transactional flows, and relationship mapping. For example, SAMS (an advanced AML screening solution) that has been built by Solytics Partners uses real-time APIs to screen entities, individuals, and even vessels against global sanction lists.
Scalability and Real-Time Processing:
Traditional financial crime solutions operate on predefined rules, which can limit their ability to catch evolving crime typologies. Cloud-native AI solutions overcome this by analyzing multiple transaction flows across different markets and generating insights almost instantaneously. For example, HSBC’s Dynamic Risk Assessment (DRA) uses Google Cloud’s AML AI to analyze transactions across multiple geographies, reducing processing times for batch analysis from one month to a few days.
Flexible Deployment and Enhanced Compliance:
Cloud-native solutions enable banks to adapt to region-specific regulatory requirements. For instance, the ability to store and process data securely in multiple regions facilitates compliance with international data sovereignty regulations. This is particularly important given the rising compliance costs, estimated to increase by 8% over the next three years.
AI for Enhanced Risk Scoring and Investigation Efficiency:
These solutions employ predictive analytics to generate risk scores, identify false positives, and prioritize alerts. Advanced solutions further incorporate network and link analysis to detect suspicious connections between customers, enhancing the accuracy and relevance of investigations. Tools like the alert management platform used by InCred, automatically scores alerts, reducing human error and improving response times.
Emerging Trends in the AI-Driven Financial Crime Detection Market
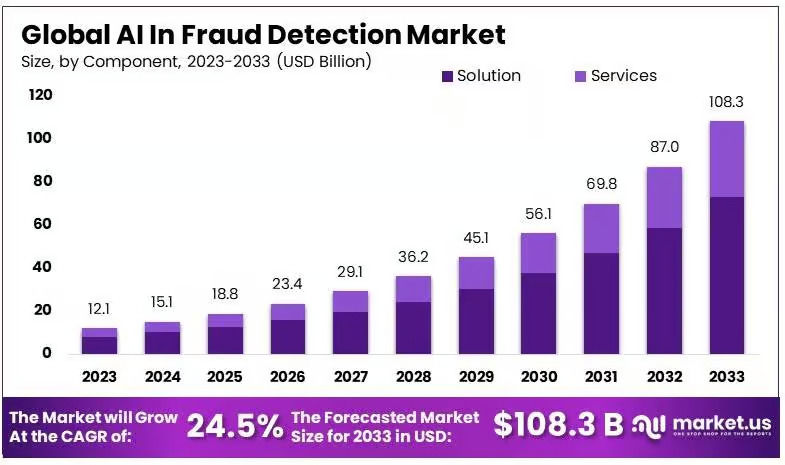
The market for cloud-native AI solutions in financial crime is highly dynamic, with both established firms and innovative new entrants offering specialized technologies. Key trends include:
Self-Tuning Models and Real-Time Adaptation:
Many financial crime detection platforms now use self-tuning models to reduce the need for manual recalibration. This reduces compliance overheads while improving the accuracy of detection in real time. Self-tuning models, which adjust based on live data inputs, are increasingly used to streamline alert generation and reduce the burden of false positives.
Broader Integration Across the Compliance Ecosystem:
New solutions are incorporating comprehensive risk management features, such as automated customer risk assessment, due diligence orchestration, and seamless integration with sanctions databases. This level of integration allows institutions to consolidate efforts across compliance silos, which previously operated independently. For example, the introduction of FinCen requirements has accelerated the integration of customer risk scoring and transaction monitoring functionalities within a unified compliance ecosystem.
Enhanced Automation in Compliance:
Automation is driving the operational transformation of financial crime compliance. By leveraging AI-driven alert management, transaction monitoring, and case management workflows, financial institutions are not only reducing manual interventions but also achieving higher accuracy in suspicious activity reporting (SAR). Automated AML solutions, such as those implemented for InCred’s clients, now process sanctions and adverse media screening with natural language processing to eliminate irrelevant information.
Benefits of Cloud-Native AI Solutions for Financial Institutions
The benefits of cloud-native AI solutions in financial crime detection are multifaceted:
Cost Reduction: Cloud-based compliance platforms provide significant cost savings by reducing the need for extensive hardware and minimizing manual interventions. The cloud infrastructure allows institutions to scale compliance operations in a cost-effective manner.
Efficiency and Accuracy: By utilizing AI to monitor vast amounts of transactional data and to analyze intricate patterns, institutions achieve higher accuracy in risk detection, minimizing the number of false positives and improving SAR conversion rates.
Enhanced Scalability and Flexibility: Cloud-native platforms empower institutions to scale their operations quickly without the burden of complex infrastructure. This agility is particularly valuable for global institutions operating across multiple regions.
Conclusion
The shift towards cloud-native AI in financial crime detection marks a significant milestone in the industry’s approach to compliance. These solutions, combining real-time data processing, scalability, and advanced ML algorithms, address the evolving landscape of financial crime with precision and adaptability. As the market continues to evolve, cloud-native AI will remain central to financial institutions' strategies to detect and prevent financial crime effectively, while also driving operational efficiencies and reducing compliance costs.
By embracing these innovations, financial institutions are better positioned to respond to increasingly sophisticated criminal tactics, and to maintain the security and integrity of the global financial system.
(The author is Managing Director at InCred Insight. Views expressed are personal.)
Comments